Artificial Intelligence (AI) has rapidly become a transformative force across various industries, and the realm of education is no exception. Before delving into the nuances of AI in education, it's imperative to understand what artificial intelligence encompasses.
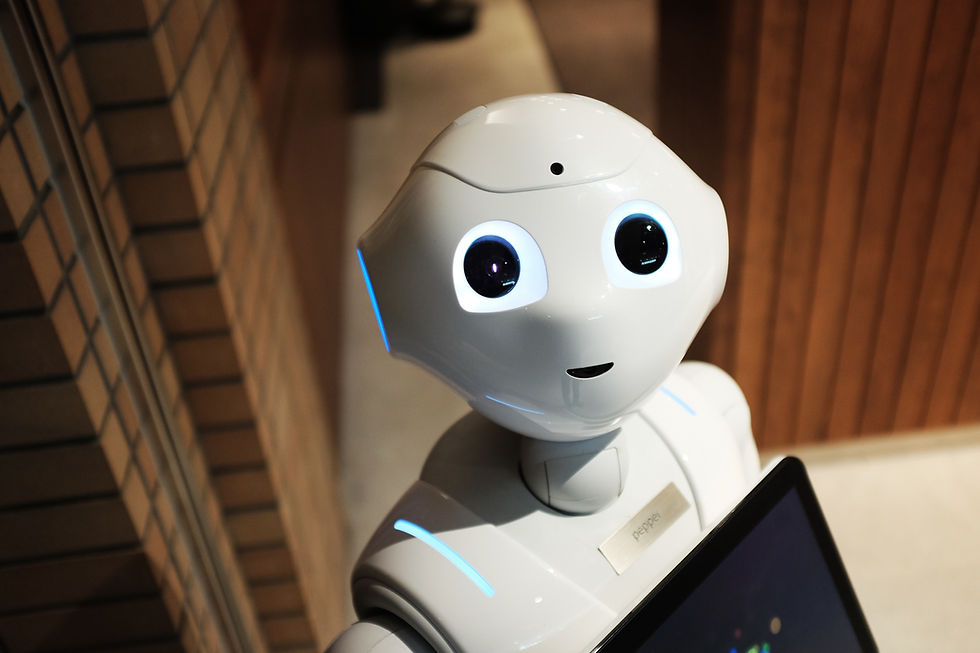
Artificial Intelligence (AI) is not just a buzzword; it's a technological marvel that is reshaping the way we live, work, and learn. At its core, AI refers to the development of computer systems that can perform tasks that typically require human intelligence. These tasks encompass a wide range of activities, from recognizing speech to making decisions, and even understanding natural language.
To demystify AI, let's break it down into two main categories: Narrow AI and General AI. Narrow AI, also known as Weak AI, is designed to handle a specific task, such as voice recognition or image analysis. On the other hand, General AI, or Strong AI, is a hypothetical form of AI with the ability to understand, learn, and apply knowledge across various domains—a level of intelligence akin to human capabilities.
The Heart of AI: Machine Learning
Now, within the expansive realm of AI, one of its most captivating facets is Machine Learning (ML). Imagine Machine Learning as a diligent apprentice eager to learn from vast amounts of data. Instead of being explicitly programmed to perform a task, the system learns from experience and improves its performance over time. But how does this learning process occur?
The Art of Learning: A Metaphor for Machine Learning
Let's think of Machine Learning as a chef learning to create the perfect dish. At the beginning of their culinary journey, the chef may not have the recipe for the ideal meal. They start by experimenting with various ingredients, adjusting proportions, and noting the reactions of those who taste their creations.
In the same way, a Machine Learning model is presented with data—ingredients, if you will. This data could be images, text, or any other form of information. The model, like our chef, begins to recognize patterns within this data. It identifies correlations, understands relationships, and refines its ability to make predictions or decisions.
The Three Pillars of Machine Learning: Supervised, Unsupervised, and Reinforcement Learning
1. Supervised Learning
In the world of culinary arts, supervised learning is akin to having a mentor guiding the chef at every step. The mentor provides the chef with labeled examples of successful dishes, explaining the key elements and flavors. The chef, in turn, learns to generalize this knowledge and apply it to create new recipes.
In Machine Learning, supervised learning involves training the model on labeled data, where the correct answers are provided. The model learns to make predictions or classifications by associating input data with the correct output.
2. Unsupervised Learning
Now, imagine our chef exploring an eclectic farmers' market without specific instructions. They have a variety of ingredients but no predefined labels or recipes. In unsupervised learning, the model discovers patterns and relationships within data without explicit guidance on what to find.
Clustering, where the model groups similar data points, and dimensionality reduction, which simplifies complex data, are common techniques in unsupervised learning.
3. Reinforcement Learning
In the culinary world, reinforcement learning is comparable to our chef experimenting in the kitchen, receiving feedback based on the flavors of their dishes. The chef learns which combinations are delightful and which need improvement, refining their skills over time.
In Machine Learning, reinforcement learning involves an agent learning to make decisions by receiving feedback in the form of rewards or penalties. The model adjusts its actions to maximize cumulative rewards, honing its decision-making capabilities.
Now that we've got a good sense of what AI really is, let's discuss the pros and cons of using AI in the field of education.
The Pros of Artificial Intelligence in Education
1. Personalized Learning
AI, particularly machine learning algorithms, can analyze the learning patterns of individual students. This analysis enables the creation of personalized learning paths, catering to each student's strengths and weaknesses. This adaptability fosters a more effective and engaging learning experience.
2. Enhanced Efficiency
Automating administrative tasks, such as grading, scheduling, and record-keeping, allows educators to focus more on teaching. This efficiency boost can lead to better use of resources and improved overall productivity within educational institutions.
3. Accessibility
AI has the potential to bridge gaps in education by providing access to quality education in remote or underprivileged areas. Virtual classrooms, intelligent tutoring systems, and educational apps powered by AI can bring learning opportunities to students who might otherwise lack access.
4. Identifying Learning Disabilities
Machine learning algorithms can analyze data to identify patterns indicative of learning disabilities or challenges. Early detection allows for timely intervention, providing students with the support they need to overcome obstacles to learning.
5. Global Collaboration
AI facilitates global collaboration among students and educators. Virtual classrooms, language translation tools, and collaborative platforms powered by AI break down geographical and linguistic barriers, fostering a more interconnected and diverse educational experience.
The Cons of AI in Education
1. Bias in Algorithms
One of the significant concerns with AI in education is the potential for algorithmic bias. If machine learning algorithms are trained on biased data, they can perpetuate and even exacerbate existing inequalities in education. For example, an algorithm might inadvertently favor certain demographic groups over others.
2. Job Displacement
As AI takes over routine tasks like grading and administrative duties, there is a legitimate concern about job displacement for educators in these roles. Striking the right balance between automation and human involvement is crucial to prevent job losses and ensure the quality of education.
3. Lack of Emotional Intelligence
While AI excels at processing vast amounts of data and performing specific tasks, it lacks the nuanced understanding and emotional intelligence that human educators bring to the table. Building relationships, understanding individual needs, and providing emotional support are essential aspects of the education process that AI may struggle to replicate.
4. Privacy Concerns
AI systems often rely on vast amounts of data to function effectively. This reliance raises significant privacy concerns, especially when dealing with sensitive information about students. Striking a balance between leveraging data for educational improvement and protecting individual privacy is a delicate challenge.
5. Overreliance on Technology
The integration of AI in education should be a tool to enhance learning, not a replacement for traditional teaching methods. Overreliance on technology may lead to a lack of critical thinking skills and creativity development in students.
Future Implications for Legislators
As the integration of AI in education continues to evolve, lawmakers and legislators must carefully consider several key aspects to ensure the responsible and ethical use of AI in educational settings.
1. Establishing Ethical Guidelines
Legislation should prioritize the development and implementation of ethical guidelines for the use of AI in education. These guidelines should address issues such as algorithmic bias, data privacy, and the responsible use of student data to prevent unintended consequences.
2. Investing in Teacher Training
Ensuring that educators are well-equipped to navigate and utilize AI tools is crucial. Legislators should allocate resources for comprehensive teacher training programs that focus on integrating AI into the curriculum while maintaining the human touch in education.
3. Addressing Equity Issues
Legislation must actively work to address and rectify any disparities exacerbated by AI in education. This includes ensuring that AI algorithms are regularly audited for bias and that access to AI-powered educational tools is equitable across different socio-economic backgrounds.
4. Encouraging Research and Development
To stay ahead of the curve, legislators should encourage and fund research into the development of AI technologies that specifically target educational challenges. This includes supporting initiatives that explore the potential of AI in addressing learning disabilities, enhancing accessibility, and promoting inclusive education.
5. Establishing Oversight Mechanisms
Legislation should establish robust oversight mechanisms to monitor the implementation of AI in education. This includes regular audits of algorithms, transparency in decision-making processes, and mechanisms to address concerns raised by students, educators, and parents.
Artificial Intelligence in education holds immense promise, offering opportunities for personalized learning, efficiency gains, and global collaboration. However, these benefits come with their own set of challenges, including algorithmic bias, job displacement, and privacy concerns. As legislators navigate the uncharted waters of regulating AI in education, a delicate balance must be struck to harness the benefits while mitigating potential risks. By establishing ethical guidelines, investing in teacher training, addressing equity issues, encouraging research, and establishing oversight mechanisms, legislators can pave the way for a responsible and inclusive integration of AI in education.
Sources:
Comments